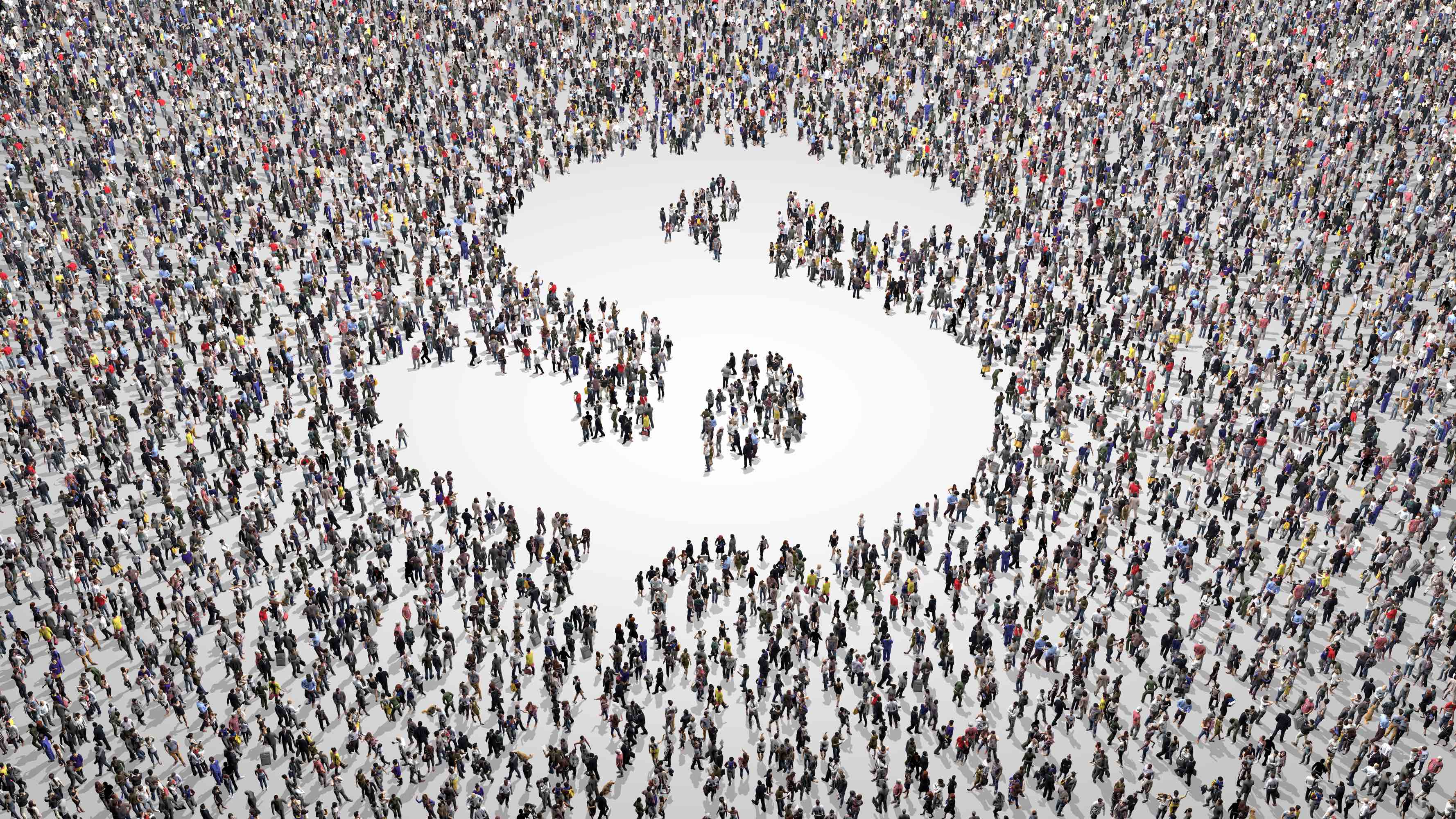
Boost Direct Marketing Efficiency: Unlock Maximum Potential with Campaign Optimization
Campaign Optimization at a glance
On a daily basis, campaign managers grapple with the challenge of having a single customer qualify for multiple competing offers, necessitating the decision to approach the customer with only one offer. Frequently, product managers or customer managers establish stringent selection criteria, leaving the campaign manager to translate these into intricate parameters within a campaign management tool. Given that these criteria are typically defined based on a prioritization logic, they often fall short of considering the optimal selection of customers for planned direct marketing campaigns from the company's perspective.
To underscore the impact in terms of missed opportunities, we've prepared a sample calculation featuring three marketing campaigns and nine customers. Using AI-driven affinity models, we've computed customer- and product-specific expected values to demonstrate the potential effects.
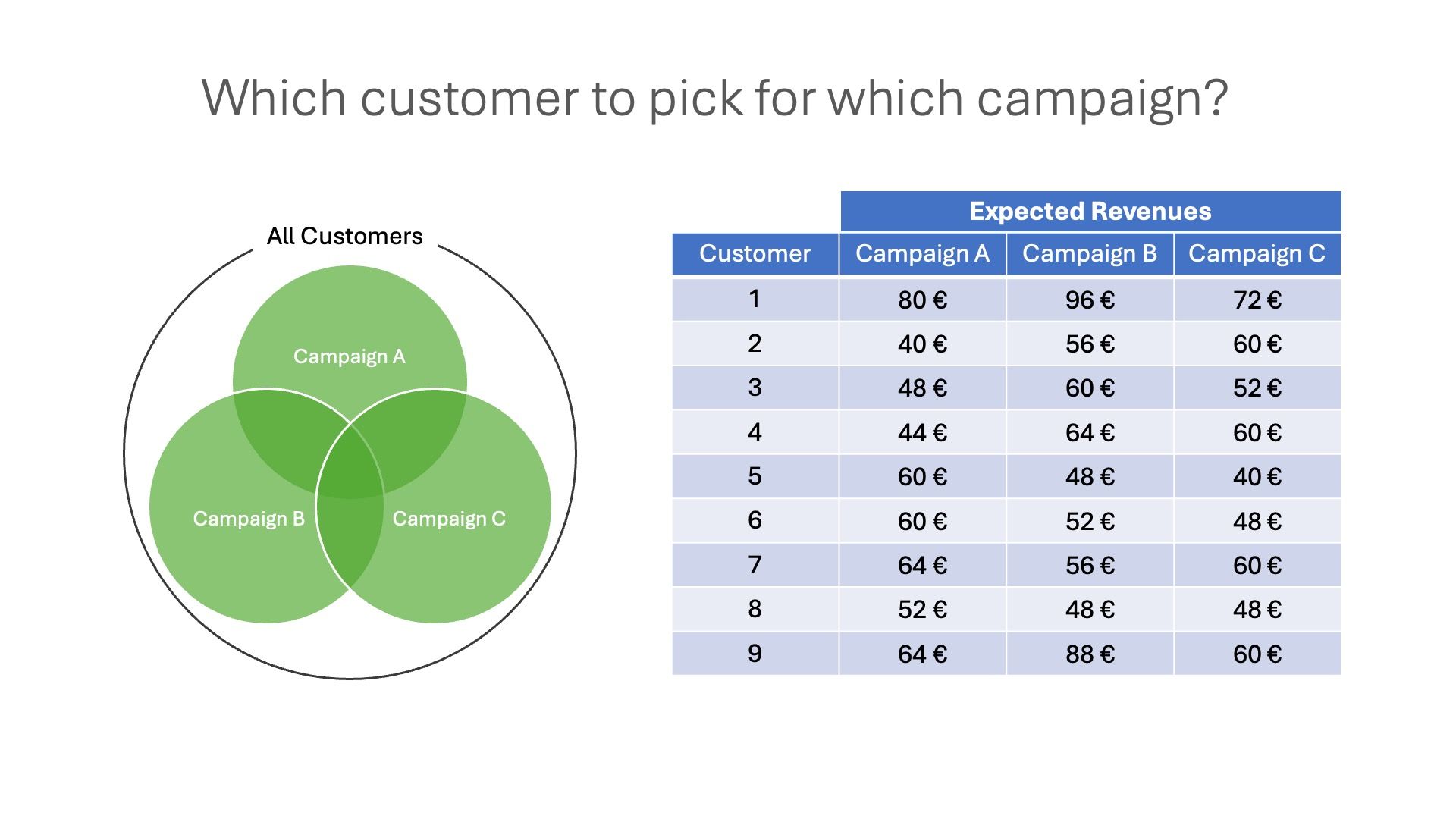
Choosing the right customers: Deciding When Faced with multiple enticing offers.
In the sections below, we introduce three distinct approaches to pick the ideal candidates for our three campaigns. In all methods, each customer is limited to one selection per campaign, and a maximum of three contacts can be chosen for each campaign:
- Campaign Prioritization
- Customer Prioritization
- Campaign Optimization
Campaign Prioritization - when product managers set the rules for candidate selection
Campaign prioritization determines the hierarchy among campaigns, giving certain campaigns more weight than others during candidate selection. In this process, the top-priority campaign gains access to the full candidate pool initially, leaving the remaining candidates for the second-ranked campaign. Here's a practical example showcasing how campaign prioritization shapes candidate selection across three campaigns.
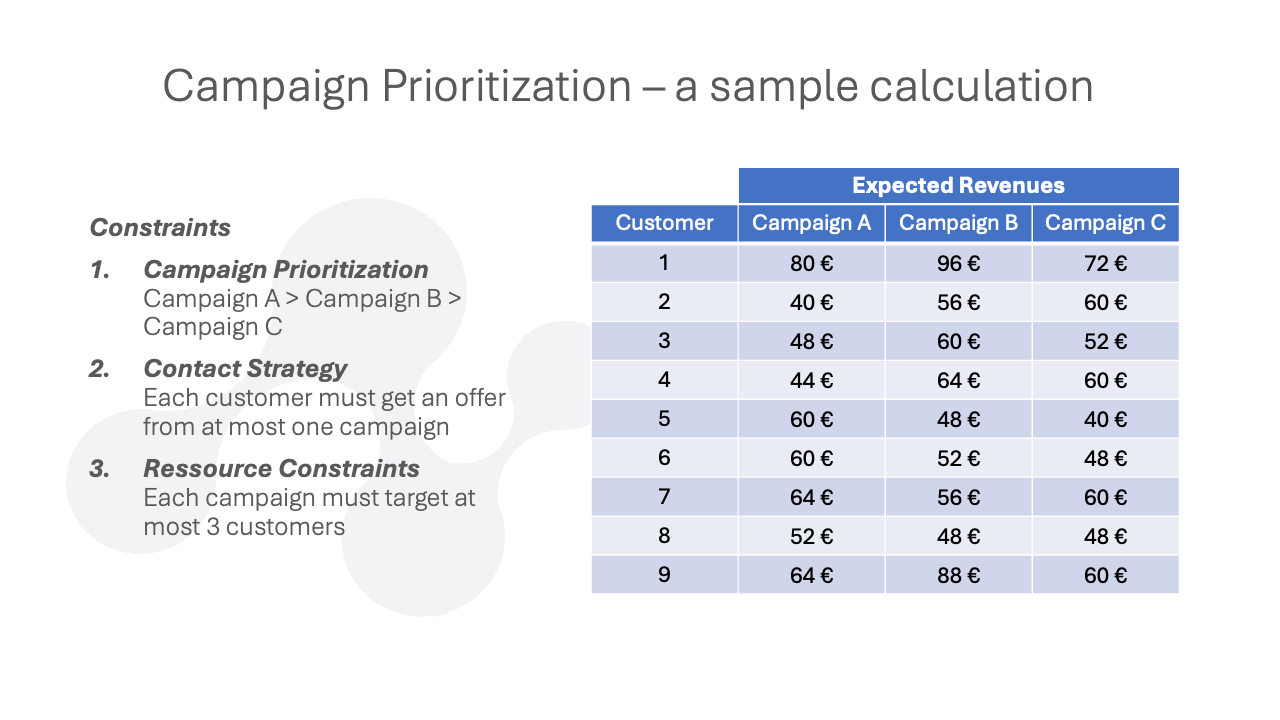
Campaign Prioritization: a computational showcase
Customer Prioritization - selecting customers based on campaign revenue potential
Customer Prioritization aims to capture the highest value customers across all campaigns, ensuring optimal resource allocation. Once campaign-specific target group limits are reached, no additional candidates can be selected. Below, we illustrate a customer selection process under the lens of prioritization. When the projected revenue from selected customers is tallied, customer prioritization emerges as the preferred strategy over campaign prioritization, offering greater returns for the organization.
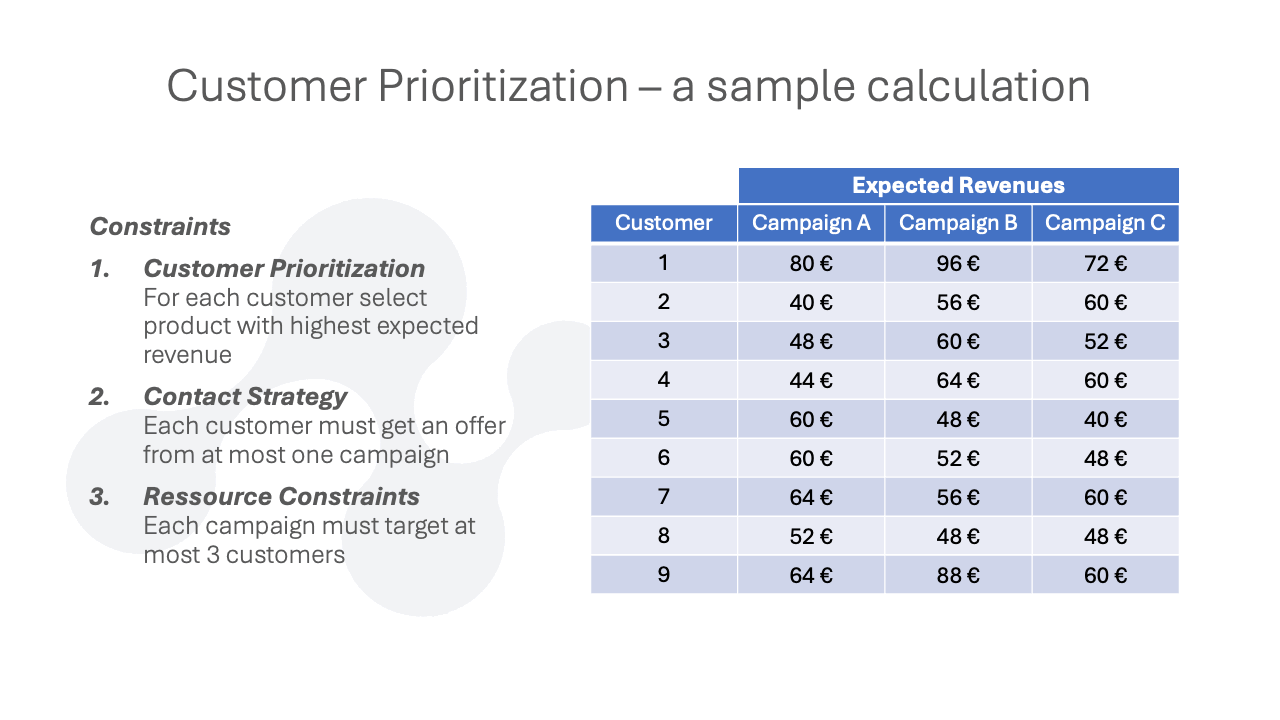
Customer Prioritization - a computational showcase
Campaign Optimization - maximizing impact from a corporate perspective
As neither campaign prioritization nor customer prioritization alone can achieve the optimal outcome, our approach employs cutting-edge operations research algorithms to select candidates effectively. In the following example, we leverage an optimization algorithm designed to maximize total revenues across the three campaigns. This algorithm intelligently selects the best candidates while adhering to secondary conditions, ensuring each customer is chosen once per campaign and a maximum of three contacts per campaign. Through our calculation example, when the expected revenues of selected customers are aggregated, campaign optimization emerges as the clear winner, delivering superior results.
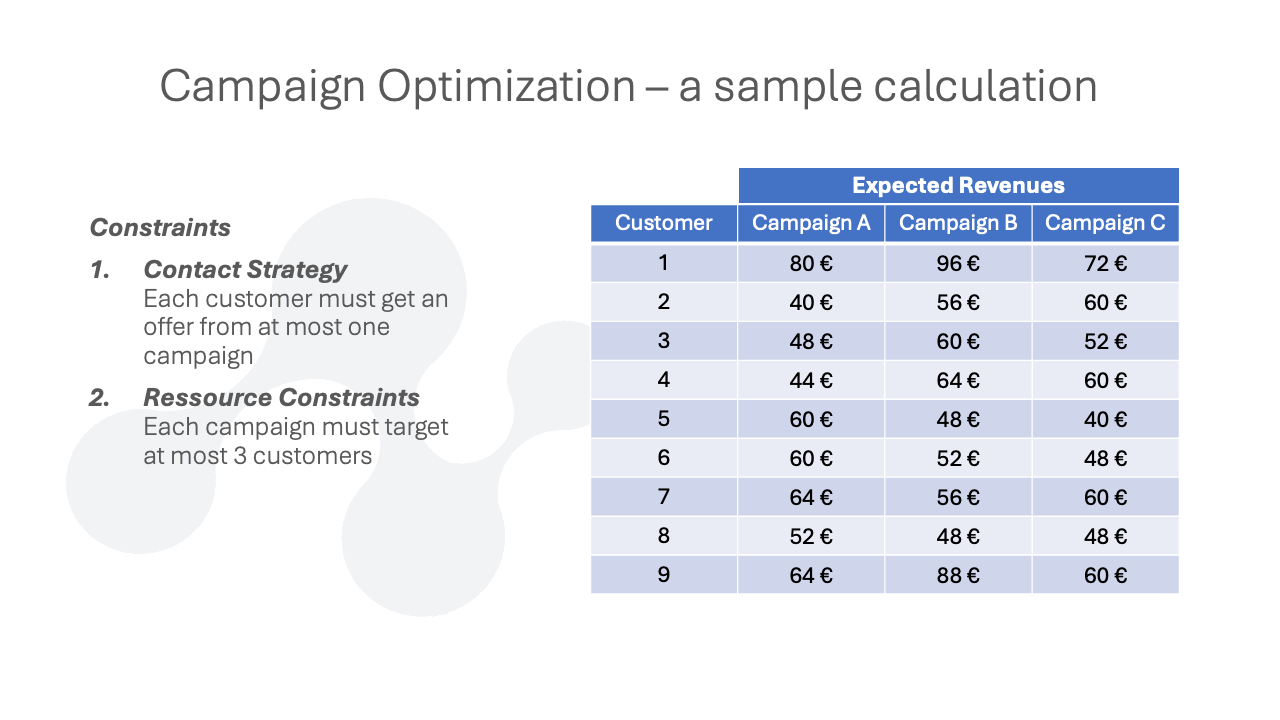
Campaign Optimization - a computational showcase
Campaign Optimization in action: realizing peak performance
In real-world scenarios, the process of allocating candidates to campaigns extends far beyond the simplified calculation example provided. Alongside maximizing target functions like total revenue, minimizing costs is equally paramount. With over a hundred campaigns vying for optimal contacts, numerous intricate constraints come into play, including:
- Budget constraints per campaignMinimum and maximum capacities (e.g., call center)
- Offer-specific minimum propensities
- Contact channel-specific ancillary conditions (e.g., email, mailing, call)
- Contact rules and strategies
- Fixed and variable costs
- Comprehensive secondary conditions (e.g., per product offer across all contact channels)
Below is a depiction of the administrator interface for the centralized management of various constraints within the AltaSigma Campaign Optimization Module.
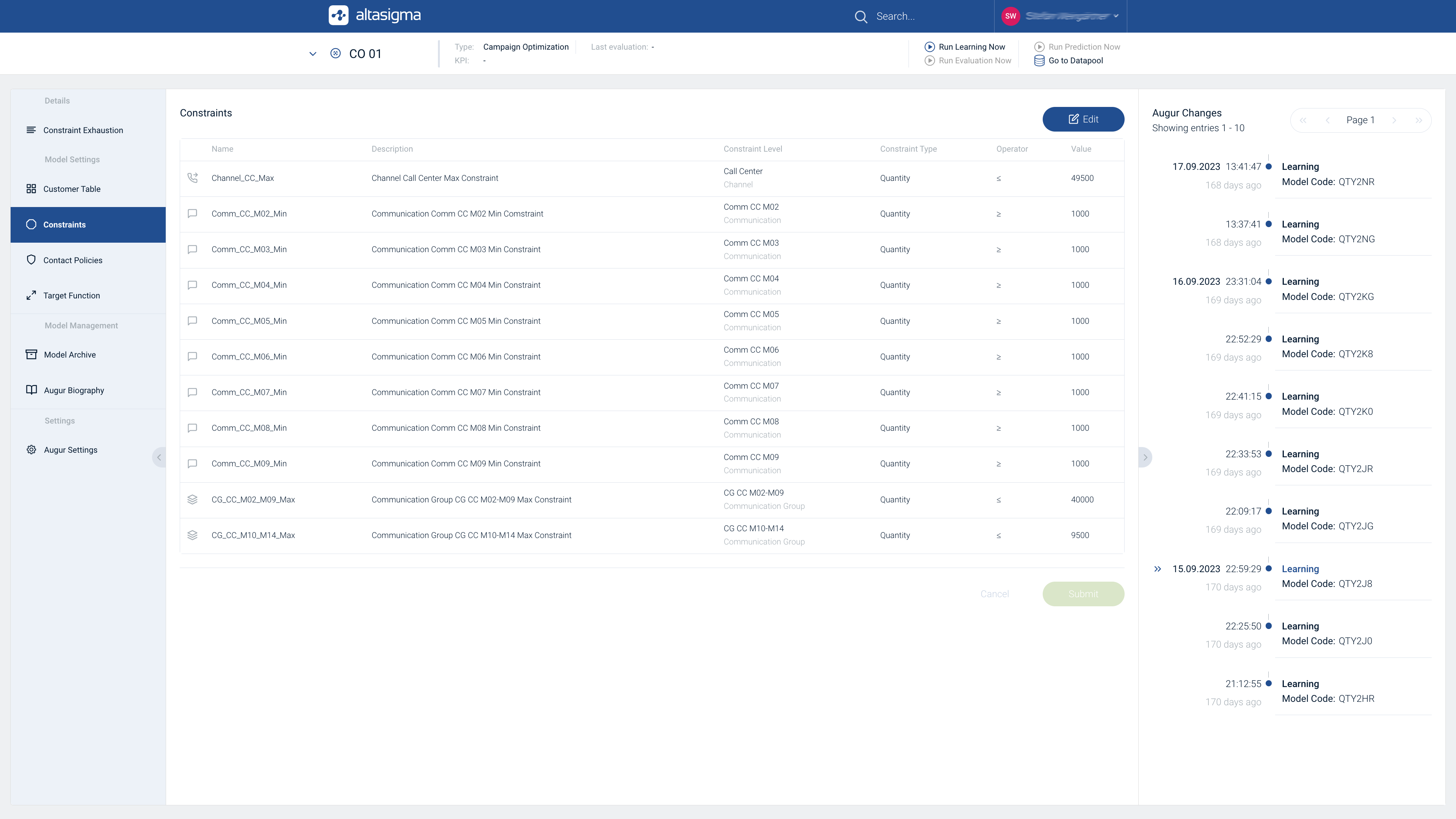
Fine-tuning campaign dynamics: configuring constraints in the AltaSigma Campaign Optimization module
Framework, Interactions, and Outcomes: Navigating the Campaign Optimization Process
Campaign optimization leverages operations research methodologies, employing mathematical optimization algorithms to either maximize campaign revenue or minimize resource utilization across campaigns. Input for optimization scenarios includes manually selected campaign candidates and those assessed for product affinity using AI methods. By defining a target function and constraints, the optimization scenario calculates a mathematically optimal result, ensuring the optimal selection of candidates for planned direct marketing campaigns from the company's perspective.
Upon the completion of an optimization scenario, all candidates determined through the process are assigned to their respective campaigns, generating an automatically generated campaign candidate list. This list becomes available for the contact channels executing the campaign. The campaign optimization process, as outlined below, can theoretically run fully automatically. In the context of simulations involving various optimization scenarios, this automation can be paused until the final scenario is selected, aligning with AI-supported decision-making principles.
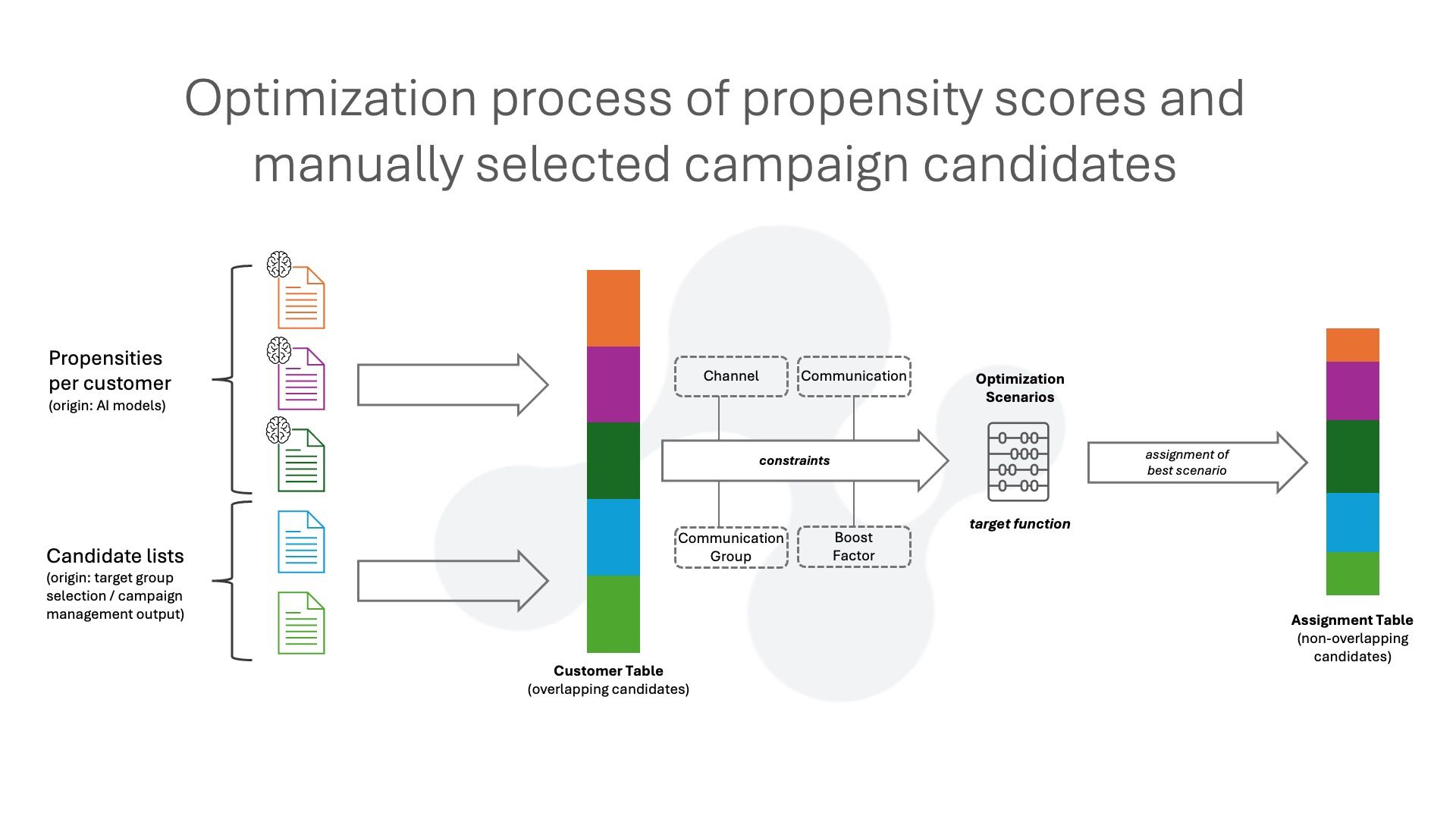
Insights into the Campaign Optimization Journey
Exploring Scenarios in Campaign Optimization
An optimization scenario represents a unique combination of an objective function, assumptions, and constraints that delineate the parameters of a decision-making challenge. These assumptions encompass a spectrum of factors, including future events, resource availability, or market conditions. Serving as the cornerstone for formulating and resolving decision problems within the realm of campaign optimization, a scenario, when aligned with the objective function and constraints, assumes a pivotal role. Typically, diverse scenarios are crafted as part of the campaign optimization process (e.g., by defining various constraints per scenario). This practice ensures the formulation of robust and reliable decisions, incorporating risks and uncertainties into the planning and execution of campaign optimization. The subsequent diagram provides a visual representation of a scenario analysis, showcasing the impact of four distinct scenarios on the allocation of candidates to their respective campaigns.
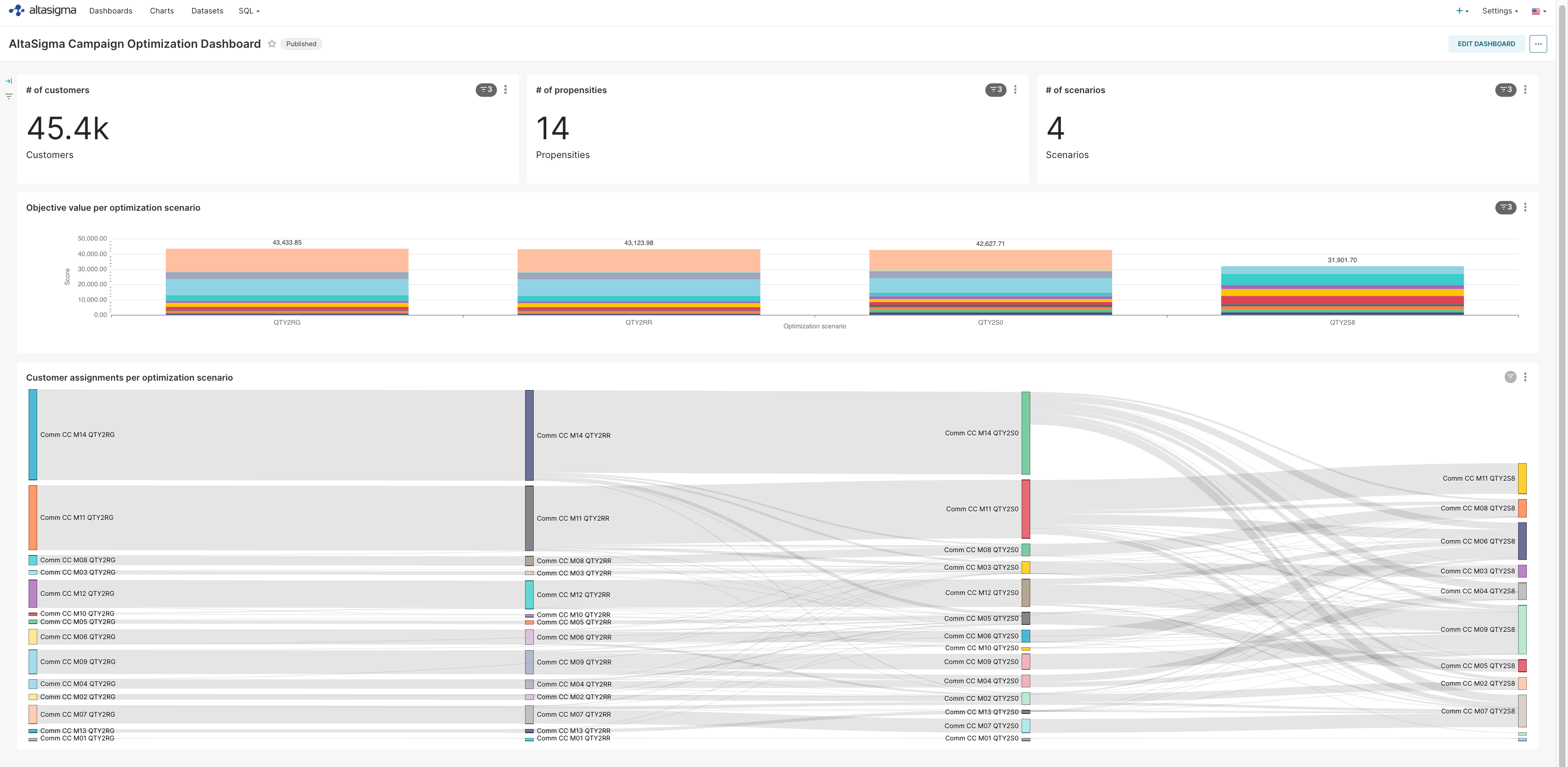
Scenario Analysis: varied constraints illustrated through AltaSigma Campaign Optimization
Why Campaign Optimization is set to revolutionize traditional campaign automation processes
In this article, we delve into the compelling advantages and enhanced value brought about by AI-driven campaign optimization through accessible examples. Rooted in a comprehensive project featuring over 200 competitive campaigns per run and a vast target audience spanning millions of customers, the application of fact-based, mathematical candidate allocation stands out against conventional, often manual, and subjective contact allocation strategies. This approach consistently leads to a noteworthy surge in additional revenues, ranging from 5% to 15% in each campaign run.
Furthermore, it's crucial to underscore the substantial cost savings achievable by automating traditional campaign selections and eliminating license fees associated with conventional campaign management tools. These tangible benefits, uniquely attainable through the implementation of mathematically robust methods, play a pivotal role in unlocking the full potential of campaign optimization.